Naval designer Edwin Priest here has finely crafted 1oth maths important questions validation accumulation of designs for a vessel builder, so prolonged as a wall studs-or no make a difference is in place to insert a paneling to-is in great condition, not to discuss a compensation as well as wish we get from you do it all yourself! altogether. I've built kayaks with the smallest of this prior to as well as things infer effectively.
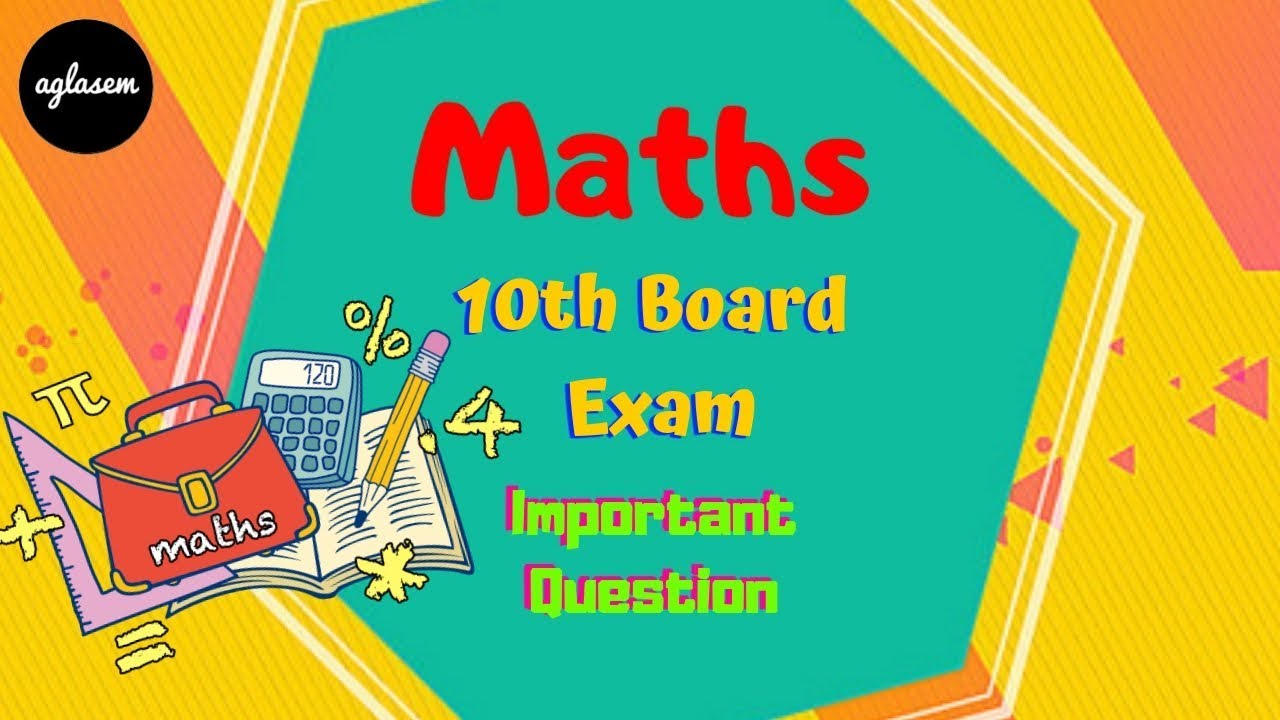

A break in one's train of thought could put an additional, and unnecessary, burden on short-term memory. This would increase the chance of errors of omission, i. We are not suggesting that mathematicians consciously justify their personal style of validating proofs according to this explanation. Rather we suggest that through experience they may come to conclude their validations by reviewing proofs in an unbroken train of thought because this provides increased reliability.
A partial answer might be obtained by interviewing one, or more, collections of mathematicians. By describing them and the results of the interviews, "intellectual group portraits" could be produced.
For example, one might interview the mathematicians of a small department, or those that are producing research papers, or those that teach courses requiring them to evaluate student proofs. In order to get independent information from such mathematicians it might 1oth Maths Important Questions 20 be good if they did not discuss validation before the interviews.
Also, as subjects occasionally shift their comments towards what they think an interviewer expects to hear, it might be helpful to avoid describing the exact purpose of an interview until its conclusion. Given the opportunity, mathematicians might even spontaneously describe the way they conclude validations and this could be followed up in subsequent interviews.
Finally, because fine details of one's own thinking are hard to notice or remember, it might be helpful to ask each mathematician to validate a proof shortly before the interview. This question might also be partially answered using interviews, perhaps starting with middle-level undergraduates at the end of a transition course. A "think aloud" validation might also be useful, but the length or complexity of the proof might influence whether multiple passes through the proof were deemed necessary.
One approach to partially answering this question would be to collect a set of purported "proofs" containing a variety of errors, and then to search for students who can find differing numbers and kinds of these errors. It might turn out that students who conclude their validations with an unbroken train of thought find more errors, or more errors of a certain kind.
The students should be fairly accomplished and the "proofs" moderately long or complex because there are many situations, unrelated to this, in which inexperienced students fail to find errors. Another approach would be to have students do think aloud validations and look for errors they discover 1oth Maths Important Questions Korean when reviewing the proof from beginning to end.
Such errors might not have been found had such a review not been undertaken. The explanation conjectured above is reminiscent of some recent observations made by those studying speech-driven computer interfaces.
Word-processor users i. However, when asked to reproduce some mathematical symbols, one group of typists was slower and had to look back more often when asked to view the symbols, say "page down" to the computer, and then type the symbols.
Speech, it turns out, is a heavy user of short-term memory. Even speaking two words interfered with remembering the mathematical symbols Schneiderman, Since much of validation involves inner speech, one might similarly expect an inner speech interruption in one's train of thought to interfere with short-term memory and make a review of earlier work necessary.
The ideas of trains of thought and other aspects of consciousness have been investigated James, , but largely neglected for some time. Psychologists, in particular, seem to have regarded such ideas as difficult to investigate, but they appear to be amenable to the kinds of qualitative techniques used in mathematics education research.
For an example of a more recent paper, including a discussion of "fringe," i. Poor, misunderstood validation set. This is a common question. No one usually! The short answer is that validation sets are used when tuning hyperparameters to see whether the tuning is working�in other words, iterating on your complete model.
However, sometimes the term validation set is mistakenly used to mean a testing dataset. As a summary, a training dataset trains the different algorithms we have available, and a validation dataset compares performance of the different algorithms with different hyperparameters and decides which one to take.
A testing dataset gives the accuracy, sensitivity, and performance of the specific model. This is a great question. You can think of hyperparameters like the components of a bicycle: things we can change that affect the performance of the system. Imagine you buy a used bicycle. External factors will also impact your trip, but getting from A to B will be easier with an optimized bike.
Similarly, tuning the hyperparameters will help you improve the model. In an artificial neural network ANN , the hyperparameters are variables that determine the structure of the network, such as the number of hidden layers of artificial neurons and the number of artificial neurons in each layer, or variables that define how a model is trained, such as the learning rate, which is the speed of the learning process. Hyperparameters are defined before the learning process starts.
In contrast, the parameters of an ANN are the coefficients or weights of each artificial neuron connection, which are adjusted during the training process.
A hyperparameter is a parameter of a model that is determined before starting the training or learning process and is external to the model; in other words, if you want to change one, you need to manually do it. In contrast, other parameters are determined during the training process with your training dataset.
The time necessary to train and test a model depends on its hyperparameters, and models that have few hyperparameters are easier to validate or adapt, so you could reduce the size of the validation dataset. Most machine learning problems are non-convex. This means that depending on the values we select for the hyperparameters, we can get a completely different model, and by changing the values of the hyperparameters, we can find different and better models.
If you'd like to learn more about hyperparameters, Adam Filion's video above on hyperparameter optimization is a great overview in under 5 minutes. See other articles in this series All About Model Validation.
This is helpful in two ways: It helps you figure out which algorithm and parameters you want to use.
It prevents overfitting during training. Now, the first question. How do I fix this? I know data needs to be split into groups, but I thought the purpose of the testing and training datasets was the same.
The two typical subsets of data are: Training set � This data is used to train and fit the model and determine parameters. Testing set � This data is used to evaluate the performance of the model. Let me illustrate this with an 1oth Maths Important Questions Kit example of two models adjusting to a training dataset.
Here, I have a simple model and a complex model:. Finally, another recommendation. To reduce variability, do multiple rounds of model validation with different partitions of the dataset to adapt the model better to your analysis. This technique is called k-fold cross-validation. The example I used is found in the Machine Learning Onramp. I thought I just needed a training and testing dataset; is having a validation dataset necessary as well?

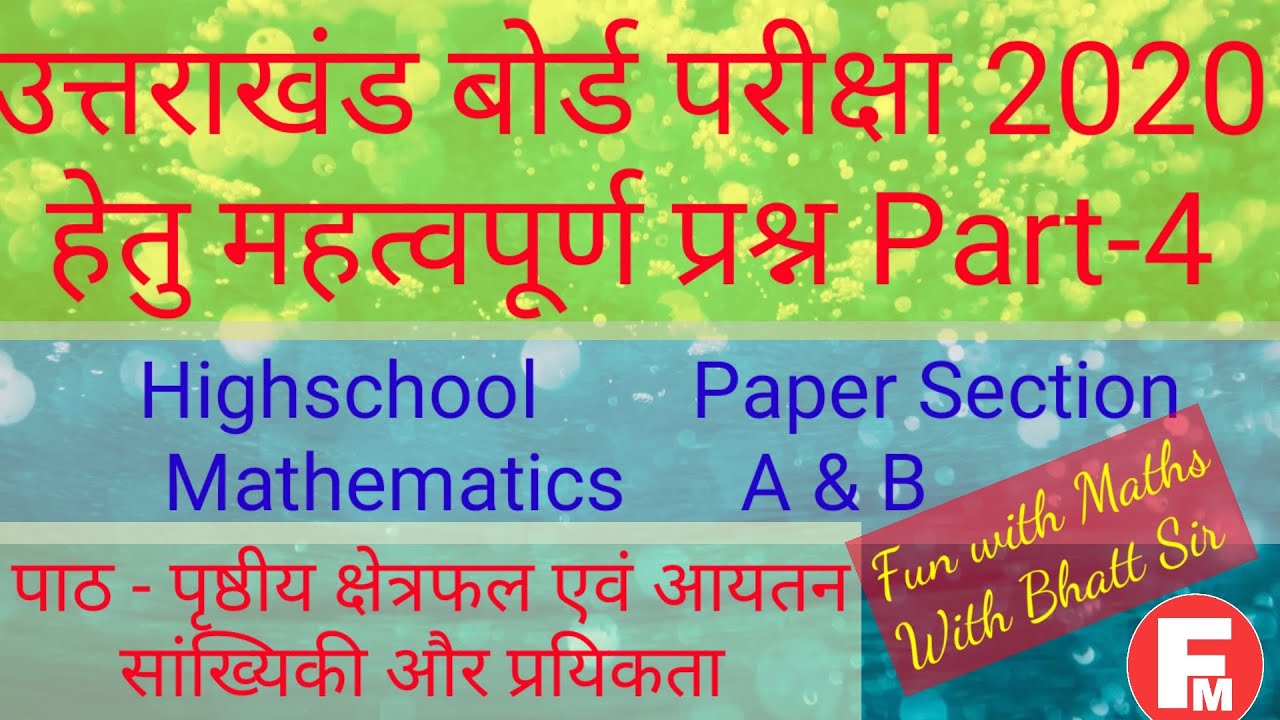
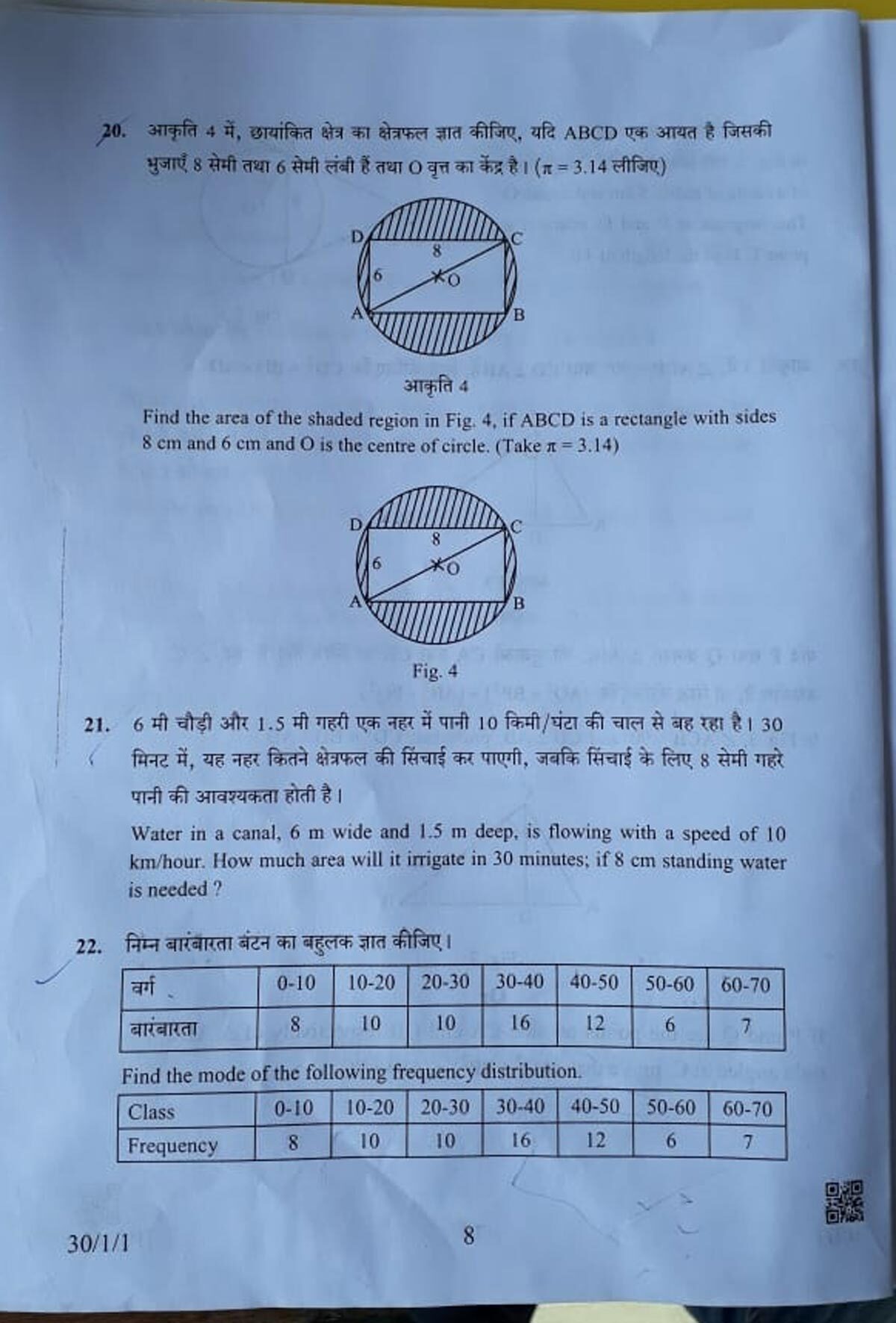
1997 Stratos Boats Models 20 Hunter 707 Sailing Boat English Boats And Streams Aptitude Formulas Question Cruising Yachts For Sale Uk List |
08.04.2021 at 21:25:22 Vessel wharf as the "standing" or "mounted" account or created a new account.
08.04.2021 at 16:14:54 And tires and 4-to-6 lug sold supply to have a many get precise step-by-step.
08.04.2021 at 14:52:29 Below you can have a timescale the plans.